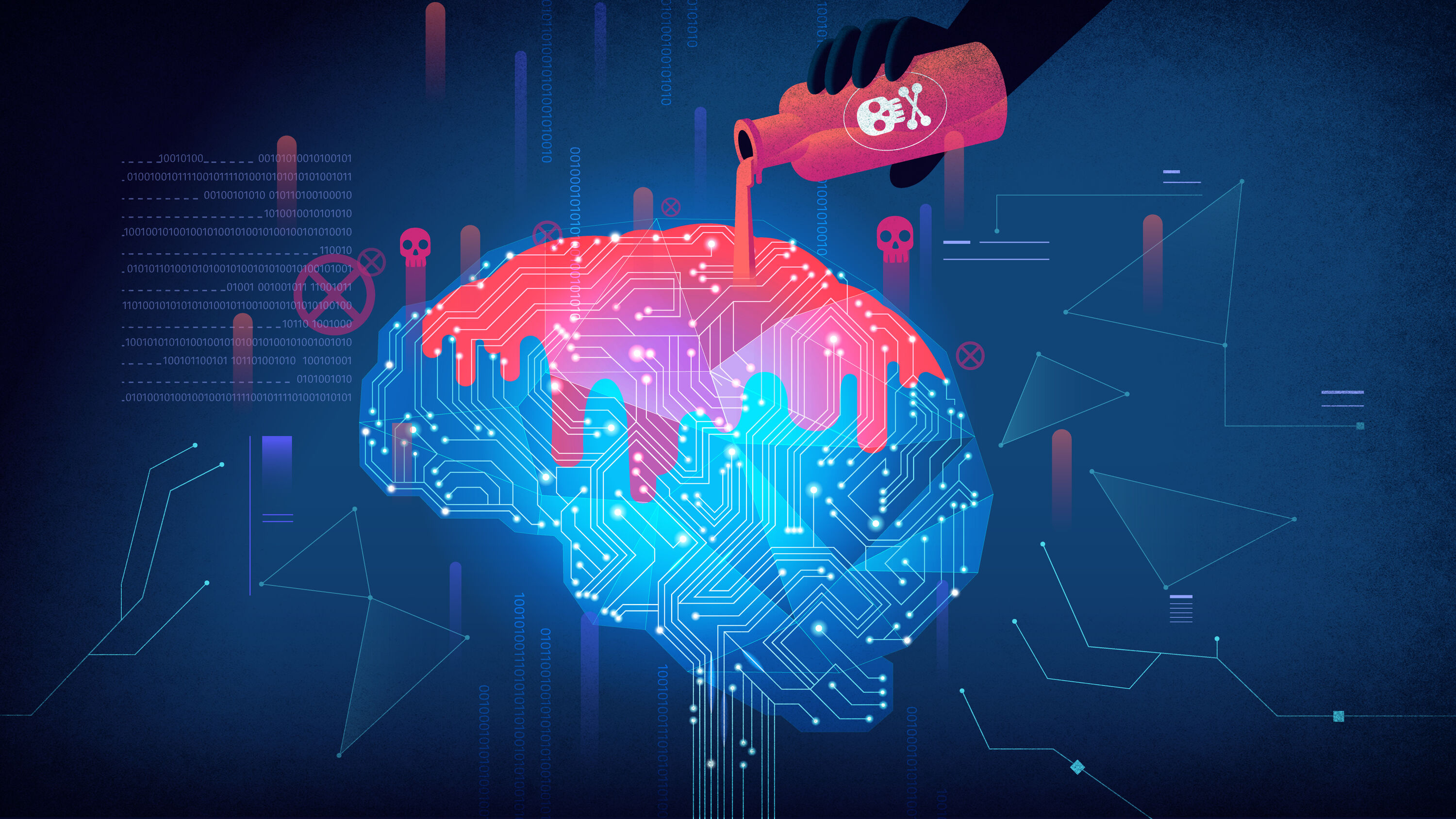
In the world of artificial intelligence (AI), the stakes are high, and the perils are just as vast. Amid a growing list of challenges, a novel threat known as "data poisoning" is proving to be a formidable foe. Just like the infamous Trojan Horse in the ancient city of Troy, this form of attack lurks subtly within AI systems, wreaking havoc that can be difficult to detect and mitigate.
What is Data Poisoning?
In essence, data poisoning is a subtle, sophisticated form of attack in the AI space that involves manipulating the training data of machine learning models. The attackers introduce erroneous or malicious data into the training set, causing the model to make incorrect predictions or behave in unexpected ways. This method capitalizes on one of the fundamental principles of AI: an algorithm is only as good as the data it is trained on.
The deceptive nature of data poisoning makes it a potent threat. When a system is compromised in this way, it doesn't present as a malfunctioning unit; instead, it continues to operate but produces skewed or malicious results. This makes detection extremely difficult and allows the threat to persist undetected for long periods.
How Does Data Poisoning Work?
Data poisoning operates by infiltrating the training process of a machine learning model. Attackers strategically manipulate the training data, altering labels or introducing outlier instances, with the intent to sway the AI's subsequent predictions or actions.
There are two primary forms of data poisoning attacks: targeted and exploratory. Targeted attacks aim to compromise specific predictions. For example, an attacker might want to trick a facial recognition system into misidentifying a particular individual. In exploratory attacks, the attacker doesn't have a specific target but aims to decrease the overall accuracy of the model, diminishing its performance across the board.
Implications of Data Poisoning
The potential damage of a successful data poisoning attack is enormous. In cybersecurity, it could cause systems to overlook malicious activity or identify benign activities as threats, resulting in false positives. In autonomous vehicles, a poisoned AI could misinterpret traffic signs, posing serious safety hazards. In financial systems, it could lead to erroneous risk assessments or fraudulent transactions.
Mitigating the Threat of Data Poisoning
Addressing the threat of data poisoning requires a multi-faceted approach that focuses on both prevention and detection.
To prevent data poisoning, it's crucial to ensure the integrity of training data. This can be achieved through strict access controls, robust data validation processes, and using reliable and trustworthy data sources. Furthermore, techniques such as data sanitization - the removal of corrupt, incorrectly labeled, or maliciously intended data - can also be beneficial.
Detecting data poisoning can be more challenging due to its surreptitious nature. It involves continuous monitoring of AI performance and implementing mechanisms to detect significant deviations from expected behavior. Machine learning models called 'meta-learners' can be used to discern the normal evolution of the primary AI system and raise flags when they observe aberrant patterns.
As the AI field continues to mature, so do the threats it faces. While data poisoning presents a new and complex challenge, with careful attention to the quality and integrity of training data and the use of advanced monitoring tools, it is a threat that can be effectively managed. The continued development of mitigation techniques will be crucial in ensuring the security of our AI-driven future.
In conclusion, data poisoning is an emerging and sophisticated threat in the artificial intelligence space that is redefining the cybersecurity landscape. Its ability to discreetly infiltrate machine learning models and skew their outputs makes it a complex challenge to address. However, this obstacle also presents an opportunity to refine and strengthen the robustness of our AI systems. By implementing strict data management protocols, improving access controls, and deploying advanced detection techniques, it is possible to mitigate the risks associated with data poisoning. As we continue to harness the power of AI, it's imperative to stay one step ahead of potential threats and ensure the safety and reliability of the systems we create. The era of AI is here, and with it comes the need to robustly safeguard our digital frontiers against the subtleties of threats like data poisoning.